and drug target discovery
Hein Min Tun and colleagues published in Nature's Signal Transduction and Targeted Therapy about a multi-platform omics analysis to get a depper insight about potential strategies for severe COVID-19 patient treatment.
Abstract: Disease progression prediction and therapeutic drug target discovery for Coronavirus disease 2019 (COVID-19) are particularly important, as there is still no effective strategy for severe COVID-19 patient treatment. Herein, we performed multi-platform omics analysis of serial plasma and urine samples collected from patients during the course of COVID-19. Integrative analyses of these omics data revealed several potential therapeutic targets, such as ANXA1 and CLEC3B. Molecular changes in plasma indicated dysregulation of macrophage and suppression of T cell functions in severe patients compared to those in non-severe patients. Further, we chose 25 important molecular signatures as potential biomarkers for the prediction of disease severity. The prediction power was validated using corresponding urine samples and plasma samples from new COVID-19 patient cohort, with AUC reached to 0.904 and 0.988, respectively. In conclusion, our omics data proposed not only potential therapeutic targets, but also biomarkers for understanding the pathogenesis of severe COVID-19.
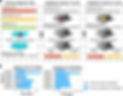
Overview of samples for multi-omics study. a Multi-omics analysis design with three datasets. The training dataset combined with severe, non-severe, and healthy controls, proteins, lipids, and amino acids were quantified in plasma and used for biomarker discovery, using random forest. The validation cohort 1 contained ten plasma samples from from non-severe and five severe patients, 25 molecules were targeted quantified for prediction evaluation. The validation cohort 2 contained urine samples corresponding to plasma samples in the training dataset, and prediction precision was further evaluated using targeted quantification. b Sample information of COVID-19 patients in the training dataset with time annotation from onset of disease to admission or from admission to discharge